Why I’ve Joined the Board of Open Humans
Gary Wolf
April 11, 2019
I’ve recently joined the board of directors of Open Humans, joining the current board along with two other new directors, Marja Pirttivaara and Alexander (Sasha) Wait Zaranek. I’m honored to be in their company, and I want to take advantage of joining the board to explain how, in my view, Quantified Self and Open Humans fit together. Both communities include many people working in science and technology who take an interest in biometric data. But this isn’t enough to define a common purpose, and in fact a much deeper connection between Open Humans and Quantified Self has developed over the last few years, as each community has approached, from nearly opposite directions, a common problem: How can we make meaningful discoveries with our own personal data?
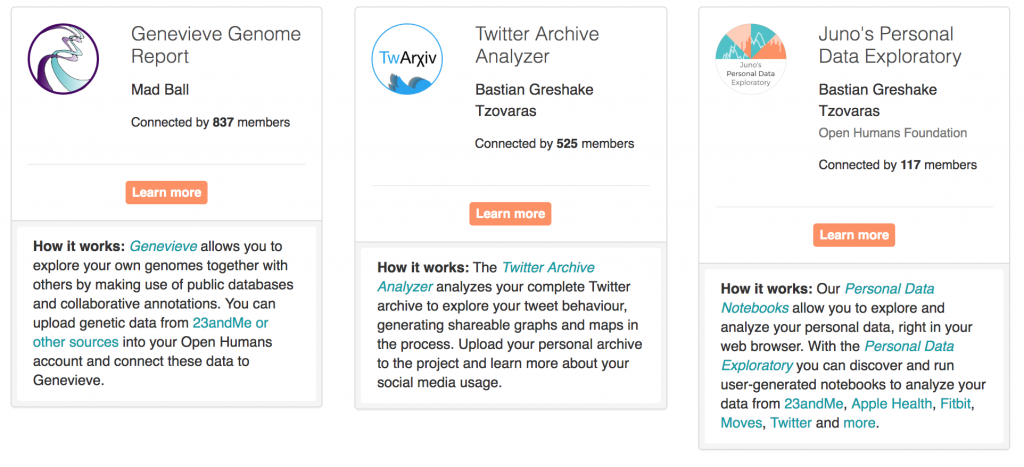
Open Humans has its roots in the Personal Genome Project, whose purpose was to supply scientists with human genomic data so that they could make discoveries more quickly. The geneticist George Church created a project to sequence the genome of individual volunteers who agreed to donate their genomic data non-anonymously, creating a common data resource. Since many important genomic questions cannot be answered with genome data alone, volunteers also shared other information about themselves. The Personal Genome Project inevitably became a somewhat more general personal data resource for science; however, with its focus on genomic data, much relevant data, including the kind of data that could be collected in daily life, remained out of scope.
When I first met Jason Bobe, who co-founded Open Humans with Mad Price Ball, he was keenly interested in this question of how to connect personal genomes with other personal data sets. Jason had worked with George Church on the Personal Genome Project. He and Mad saw Open Humans as an analogous effort, but one that would allow volunteers to contribute any kind of data. The Personal Genome Project was now a decade old. Perhaps, with deep personal data sets to work with, scientists could deliver on the promise of genomics to revolutionize medicine, a promise that had been long frustrated by the complexity connecting genomic data with real world outcomes.
I understood the goal. A few years earlier, I’d written a long Wired story about the taxonomic collaboration between Daniel Janzen and Paul Hebert. Janzen, along with his other accomplishments, was among the world’s most knowledgeable field biologists. Hebert had developed a genomic assay that promised to identify animals using an extremely small region (about 650 base pairs) of their mitochondrial DNA. Hebert was confident in his technique, but needed to prove its utility. How could the genomic data he was collecting be paired to real world ecological knowledge? At their field station in the Guanacaste Preserve in Costa Rica, Janzen and his partner Winnie Hallwachs, along with their students and colleagues, collected hundreds of butterflies and moths, identified them, snipped off a leg, and shipped it to Guelph, a city in Canada, where Hebert ran the sequence. Slowly, painstakingly, they connected the genomic data to the real world data. More than just proving that Hebert’s technique worked, they also brought a new degree of resolution to the ecological picture; showing, for instance, that individual specimens, though visually almost identical as adults, may belong to distinct evolutionary clades and feed on different plants. In my first conversations with Jason, I saw this as how Open Humans should work. It promised to provide the “field biology” for the genomic studies of the Personal Genome Project.
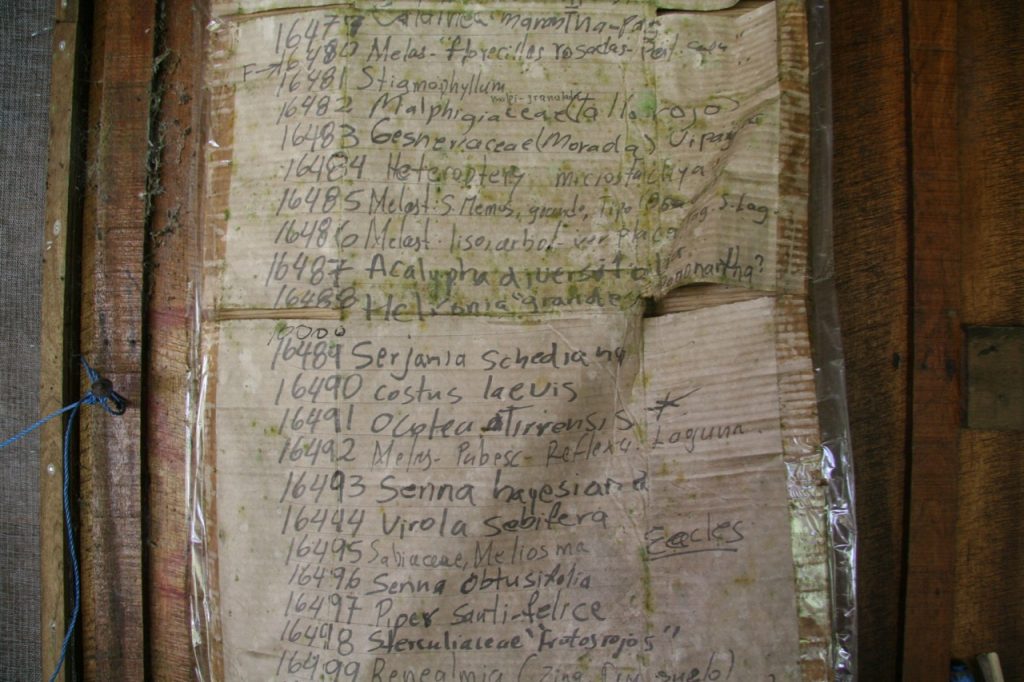
Unfortunately, as attentive readers, link followers, and experts in the history of overconfidence in science may already have realized, there’s a pretty serious flaw in my analogy. Paul Hebert was using the genome to distinguish strands in evolutionary history, mostly at the level of species. He wanted to know, given a leg, what kind of creature it was from. Answering relevant health questions requires understanding the world at a far more detailed level, down to extremely small differences among individuals of the same species. The trick that Hebert used is never going to work; and, for many of the health related questions we care about, nobody knows the tricks that will work. Fifteen years after the launch of the Personal Genome Project, it continues to supply data resources to basic science, but its relevance to medicine remains mostly a promise.
In the Quantified Self community the focus has always been on individual discovery: How can we learn about ourselves using our own data? Many of the questions addressed by people doing their own QS projects relate to health and disease. Browse the archive of Quantified Self Show&Tell presentations and you’ll find projects on Parkinson’s disease, diabetes, cognitive decline, cardiovascular health, depression, hearing loss, and many other health related issues. The kind of “everyday science” practiced in the Quantified Self community can be understood as being the opposite of the genome-wide association studies. Instead of finding small, telling differences among groups of people, the everyday science of the Quantified Self finds large effects within a single person who is both subject and scientist.
This comes with its own kinds of difficulties. People doing Quantified Self projects related to health face a number of discouraging barriers, including lack of access to their own data and medical records, bureaucratic roadblocks and exorbitant costs in ordering their own lab tests, problems in acquiring the requisite domain knowledge to test their ideas and interpret their data, and – perhaps most discouraging to people who are dependent on medical professionals for some aspect of their care – lack of recognition in the health care system that self-collected data can be useful for making decisions about treatment.
In the 11 years since Quantified Self started, participants have tried many different ways to overcome these barriers, both individually for their own projects and systematically through creating tools and advocating for better policies. One of the lessons from this work is that while the focus of self-tracking projects is typically on individual learning, the methods required to make sense of our data often require collaboration. Existing systems are not designed to provide support for the kind of highly individualized reasoning we do; therefore, we have to build a new system. Key requirements of this new system include: private, secure data storage; capacity to integrate data from commercial wearable devices; fine-grained permissions allowing sharing of particular data with particular projects, and withdrawal of permission; capacity for ethical review both to protect individual participants and to enable academic collaborations.
Two years ago, we organized our first participant-led research project in the Quantified Self community. A group of about two dozen of us measured our blood cholesterol as often as once per hour, exploring both individual questions about the patterns and causes of variation in our blood lipids and a common group question about lipid variability. We had a pressing need for some collective study infrastructure, but there was no available tool that worked for our needs. We took a DIY approach and at the end of the project we’d learned a tremendous amount both about our own varying cholesterol and about the process of self-directed research. (Our paper, “Approaches to governance of participant-led research,” has recently been published in BMJ Open. Our paper on our collective discovery about lipid variability, “Free-Living Humans Cross Cardiovascular Disease Risk Categories Due to Daily Rhythms in Cholesterol and Triglycerides” has recently been published in the Journal of Circadian Rhythms.)
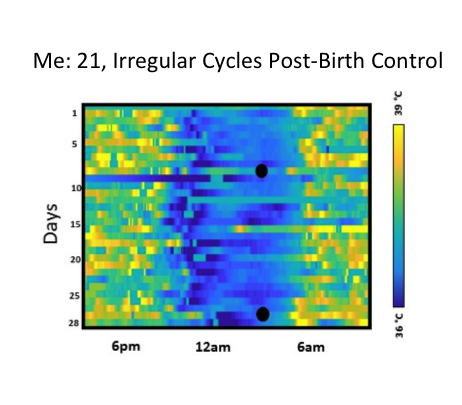
At the conclusion of our study, one of the participant organizers Azure Grant, decided to press ahead with another participant-led study on ovulatory cycling. Azure had already presented a self-study on using continuous body temperature to predict ovulation at a Quantified Self conference. Now, she wanted to organize a group of self-trackers to try something similar, but integrating newer measurement tools to acquire higher resolution data. Among these tools was the new version of the Oura ring, which offered body temperature, heart rate, and sleep data. This idea put new demands on our study infrastructure. Thanks to generous collaboration from Oura engineers, we could offer participants access to detailed data from their rings. But how could this data be stored privately and controlled by each individual, while also being available using fine-grained permissions to their fellow participants and study organizers? How could this data be integrated with other data types they might decide to collect during the project? Where was there infrastructure for a “field biology” of the self?
We turned to Open Humans. The personal reasons were as important as the technical ones. Mad Ball, along with her work leading Open Humans, is a long time participant in the Quantified Self community, who has consistently advocated for non-exploitive approaches to handling personal data, and has contributed the results of her own self-directed research. (See Mad’s recent talk on “A Self-Study Of My Child’s Genetic Risk.”) And Bastian Greshake Tzovaras, the Open Humans research director, quickly proved to be an extremely sensitive and skilled collaborator. Bastian co-founded openSNP, a grassroots effort that outgrew Personal Genome Project by supporting citizen science participation. (Currently, there are more genotyping datasets publicly shared in openSNP than all other projects in the world combined.)
With help from Mad and Bastian and the Open Humans infrastructure, we built our next stage study workflows with encouraging speed and harmony. Fundamentally, we found ourselves aligned on the core idea that research processes designed around personal data sets should be built to protect individual agency, even where this requirement creates friction for academic collaborators. The rarity of this commitment may only be obvious to those few people who have gotten painfully deep into the workflows of study infrastructure. (And I recognize that a post of this length that is this deep in the weeds can have very few readers!) But, in a way, that’s one of the beautiful things about this stage of building a new knowledge infrastructure. We’re far into it enough to have evidence that we’re on the right track. But we’re still close enough to the beginning that each step is a significant contribution and a potential model to build on.
I very much hope that over time – and the sooner the better – our shared ideas about individual agency and everyday reasoning are embodied in tools and policies that are so commonplace that no single organization is responsible for them. But for now, it’s impossible not to recognize that Open Humans is an indispensable resource, defining an approach that needs to be developed and expanded, and managed by a team that has deep insight into the challenges and potential of participatory science. I look forward to building more connections between our two communities.
Learn more about Open Humans here: https://www.openhumans.org/